Facing up to the challenge of behavioural observation in infant hearing assessment
The ability to assess detection and discrimination of speech by infants has proved elusive. Dr Iain Jackson and colleagues from our Manchester BRC Hearing Health theme discuss how new technologies and fresh approaches might offer valuable insight into young infants’ behavioural responses to sound.
Finding out whether an adult can hear something can often be as straightforward as simply asking them. Getting the same information from an infant is considerably less straightforward. However, obtaining information about an infant’s ability to detect and discriminate between sounds is crucial when making management decisions. This is especially important if we are to realise the benefits of newborn screening and early intervention.
Applying new technologies to infant audiometry
As part of our Manchester BRC research, we are currently looking to combine automatic facial recognition, head tracking, and eye tracking, along with state-of-the-art machine learning algorithms, in order to be able to detect when an infant hears a sound.
Infants excel at sending signals with their faces – all caregivers are acutely aware of how easy it is to recognise when an infant is unhappy! In addition to clear and obvious signals, like crying, there might also be other valuable markers we can capitalise on. Facial recognition software can detect and track a wide range of facial behaviours, from broad, global features such as head-turns, down to more subtle changes in individual features or areas of the face. For example, infants may raise their eyebrows ever so slightly when they hear a sound, or smile, or frown, or let their mouths fall open slightly. Whatever the response might be, if one or more responses occur frequently enough, it will provide a pattern for the algorithm to recognise, and thus provide a signal for researchers to measure.
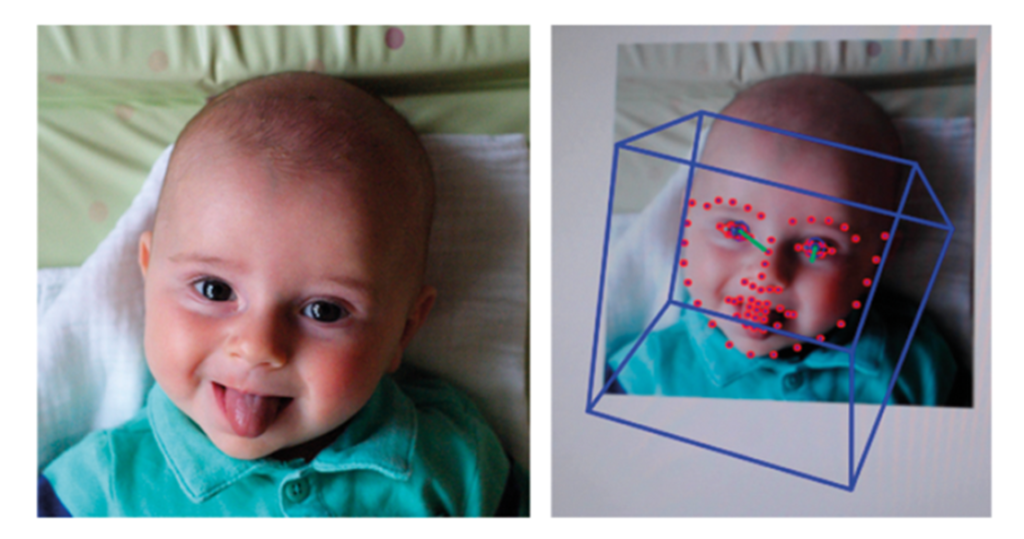
Example of the automatic detection of facial features. Here, open-source software captures both the overall head position (blue box) and facial features (red dots) in a video of an infant.
In this approach, we take video recordings of many infants’ faces and capture a range of examples of the spontaneous expressions and movements infants commonly make in the absence of sound. We also take video recordings of whatever their faces do when we play sounds. We can then use facial recognition software to map a number of points to the faces in each scenario. Finally, we can ask a computer to look for common patterns in the points, and, if patterns are found, whether they are different enough to tell the two scenarios apart.
If differences can be reliably detected between responses which occur when infants do or don’t hear sounds, or when they hear differences between sounds, then we can begin to use this approach to predict a wide range of clinically-relevant responses in individual infants, such as the detection of conversational speech, discrimination of speech sounds, identification of minimum response level, and so on. Such a system would provide hugely valuable insights into infants’ hearing very efficiently, cheaply, and quickly. It would require minimal specialist equipment, no equipment to be in contact with the infant, and could be conducted by a single researcher or clinician.
Where might improvements in technology lead us in the future?
In addition to the success of lab-based eye tracking paradigms, recent work suggests that infants’ attention can be measured by webcam video of their eye movements, and that even detailed eye tracking can be performed via an ordinary webcam. Similar success with our facial recognition approach would open up the possibility of remote, tele-audiology assessment of infants in their own homes, helping to reduce obstacles for hard-to-reach populations and offering the flexibility that caregivers frequently require. Another possible development is that tests become increasingly tailored to individuals. Automation of the delivery of the test allows for real-time, response-contingent, selection and presentation of the stimuli we use to elicit responses. Content of stimuli could easily incorporate material directly relevant to each individual infant, such as images of caregivers’ faces or samples of caregivers’ speech, in order to maximise infant attention and the likelihood of a response.
The content of this article is taken from an article originally produced for ENT & Audiology News. Read the full article.